As humans, we are prone to making mistakes, but when an AI (artificial intelligence) system stumbles upon an error, is it forgiven or forgotten? As it turns out, they are definitely not forgotten. AI systems generate solutions based on the training data they are given, but if the training data contains biased human decisions or reflects historical or social inequities, the produced results will be inaccurate and biased. Sometimes, even when sensitive variables like gender or race are removed, AI can still have biases.
Bhavini Kumari & Stuti Mazumdar - May 2023
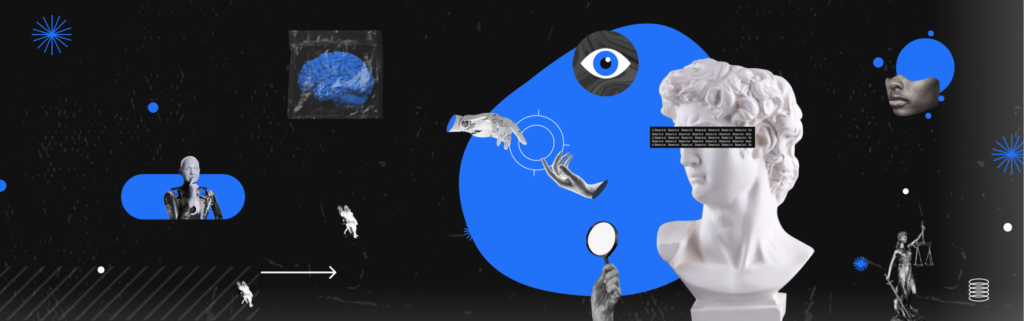
For example, let’s say that a company uses an AI system to manage its hiring operations. A specific sample was used to train the system. Assume that female candidates are underrepresented in the sample based on the last 20–30 years of training data. Even if the company cleans the input data of any gender, cultural, or racial bias, the AI algorithm will make hiring decisions based on the original sample training data, which will discriminate against female hires in comparison to their male counterparts. The consequences of these bias-driven errors could cost the company not only its reputation but also a potential discrimination lawsuit.
As more businesses look to integrate AI into their operations, the need to address and correct data biases grow exponentially.
Why should we be concerned about addressing AI biases?
Biases in artificial intelligence can have serious consequences for individuals, groups, and society. AI systems that have been trained using biased data or algorithms have the potential to perpetuate and amplify existing social, economic, and cultural biases.
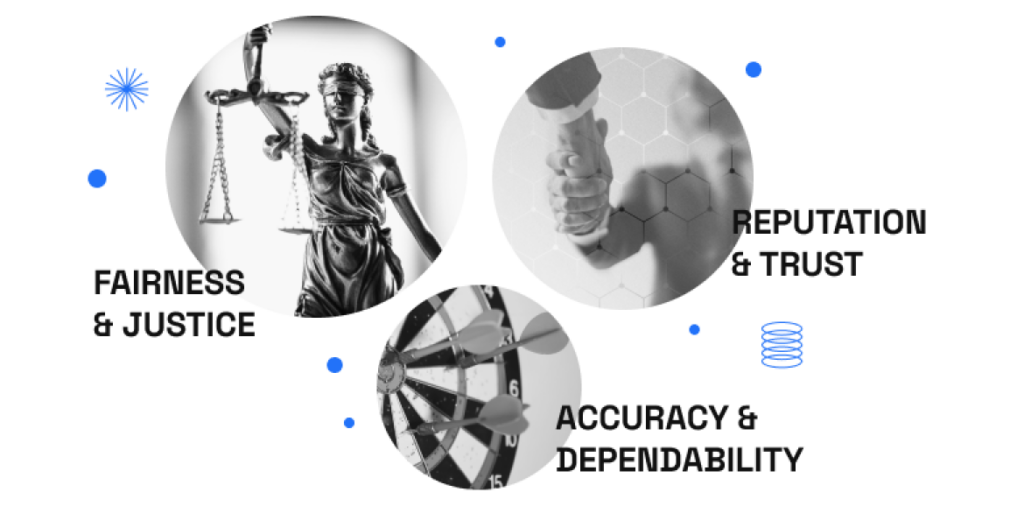
Addressing AI biases can promote innovation and progress by enabling the development of more precise and trustworthy AI systems that benefit society.
Biases in AI
Cognitive bias is the way that a person’s beliefs, feelings, and experiences affect how they make decisions or judge things. It has to do with how our brains process information. Keeping this in mind, we can name a few cognitive biases that people unintentionally add to AI systems, which severely limit how well intelligent machines can work.
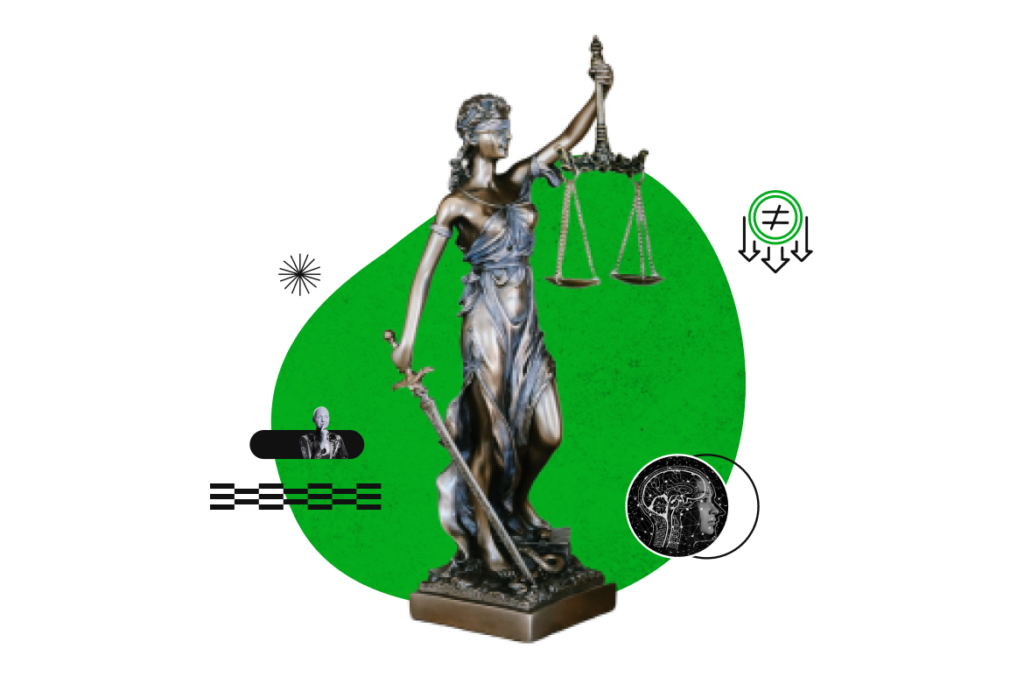
1. Systemic Biases
Historical bias: This refers to biases that have become established in society and culture over time and are mirrored in the data used to train and evaluate AI systems. If previous data used to train a hiring algorithm was biased against specific categories of people, the algorithm may perpetuate those prejudices in future hiring decisions.
Institutional bias: This refers to biases built into specific institutions’ procedures and practices seen in the design, implementation, and evaluation of AI systems. If a law enforcement organization, for example, extensively depends on predictive policing algorithms that have been demonstrated to disproportionately target minority populations, this could be an example of institutional bias.
Data bias: Biases can occur because of the quality, quantity, or nature of the data used to train and assess AI systems. For example, if a facial recognition system is trained on a dataset with primarily light-skinned people, it may be less accurate at recognizing people with darker skin tones.
Algorithmic bias: This refers to biases that are incorporated into algorithms and can result in unfair or discriminating results. For example, if a job evaluation algorithm offers better scores to applicants who attended famous institutions, this could disproportionately benefit individuals from wealthy backgrounds, perpetuating class inequities.
Deployment bias: Biases in the deployment or use of AI systems can occur due to factors such as context, environment, or human behavior. For example, if an AI system developed to assist doctors in making diagnoses is utilized by doctors in affluent areas, it may be less successful for patients from lower-income neighborhoods who may have distinct health problems or risk factors.
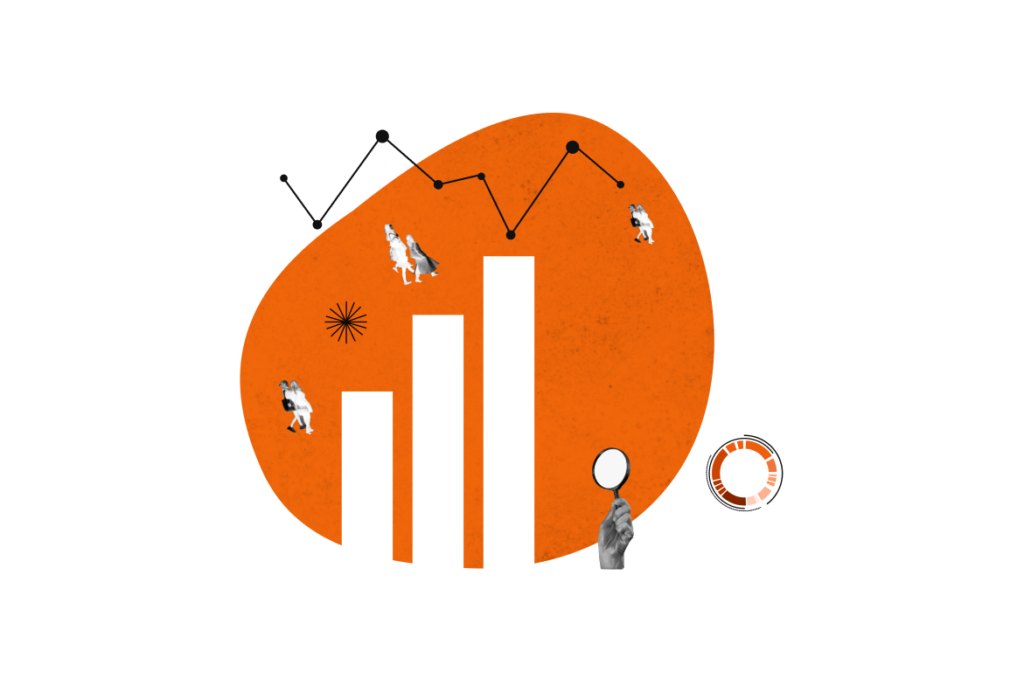
2. Statistical Biases
Sampling bias: This occurs when the data used to train an AI model is not representative of the population it is intended to serve. For example, if an AI system is trained on data from a particular region or demographic group, it may not generalize well to other regions or groups.
Measurement bias: This occurs when the model measures or categorizes data in a biased manner. A model that categorizes people based on their ethnicity or color, for example, may perpetuate existing biases and discrimination.
Confirmation bias: This occurs when the model confirms or reinforces existing biases in the data rather than challenging or correcting them.
Selection bias: This occurs when a model selectively includes or excludes certain data or features, resulting in biased results.
Recall bias: This occurs when the model is more likely to remember or prioritize certain data over others, leading to biased outcomes.
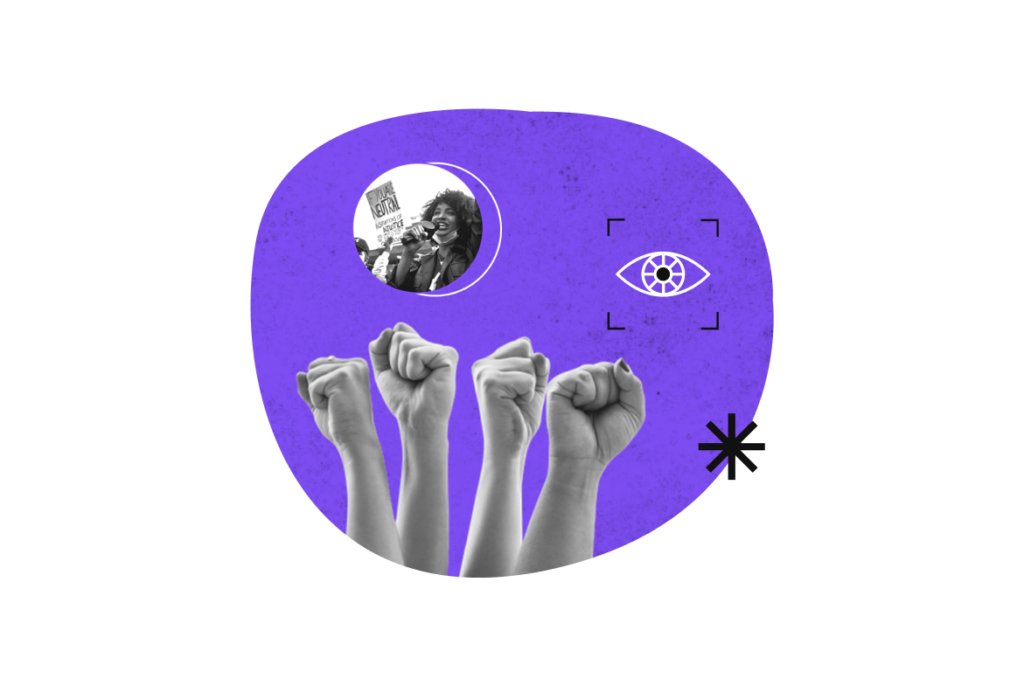
3. Human Biases
Confirmation bias: This is the tendency to seek information that confirms our existing beliefs or assumptions while disregarding or dismissing information that contradicts them. This can be seen in AI when designers or users of AI systems selectively interpret or accept outcomes that support their beliefs while rejecting or downplaying results that question them.
Anchoring bias: This is the inclination to base decisions too heavily on the first piece of information encountered, even if it is irrelevant or inaccurate. This can happen in AI when AI system designers or users place too much weight on original data or assumptions, resulting in erroneous or incomplete findings.
Availability bias: This is the bias to exaggerate the possibility or significance of occurrences or situations that are easily recalled or vivid in memory while underestimating those that are less salient or memorable. In AI, this can lead to an overemphasis on readily available data or patterns, even if they do not represent the whole spectrum of important aspects.
Framing bias: This is the tendency to be persuaded by how information is presented rather than its content. This can happen in AI when the way data is classified, labeled, or presented affects the findings obtained or the decisions made based on those results.
Group bias: This is the tendency to prefer one’s own group or identity over others, resulting in unequal treatment or chances for persons or groups viewed as distinct. In the case of AI, this can emerge as bias in the data used to train or evaluate AI systems, as well as bias in the decisions made based on those systems.
While AI biases must be weeded out for the system to become more intelligent, we are still a long way from having completely bias-free algorithms and datasets. We can, however, shorten the distance by working to improve, optimize, and refine them.
Furthermore, addressing bias in AI and its importance is only one aspect of the overall picture. How can we keep these systems in check? That is a thought we would like to leave you with.