This post is a sequel to an earlier one titled ‘UX in the times of AI: Designing with Bots’. In this post, we will look at a few philosophical aspects of cyber consciousness, collective intelligence or machine intelligence and the design considerations that must be looked at in conjunction with trusting this technology.
Hari Nallan & Symran Bhue - August 2017
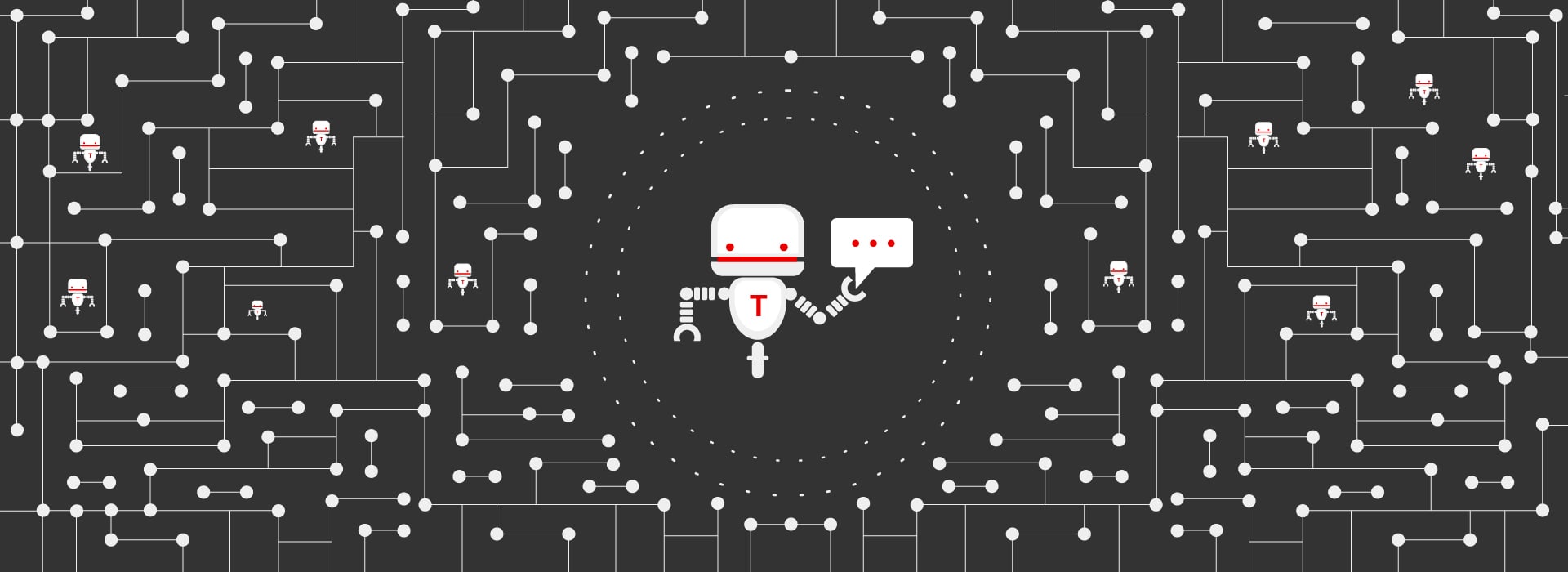
Humans have a natural disposition to trust. People have a tendency to trust other people as friends, colleagues, service providers; People trust brands and organizations for their products and services as well as trust situations where they are consuming that product or service. There are many theories on colors, words, personality types, facial features and voice quality that can evoke trust in people. In essence, people trust where there is a human element attached to it, so how close to human artificial intelligence feels will be one of the determining factors in whether it will be trusted.
In our previous post, we touched upon human and AI interactions and humanized user experiences in reference to bots with personas. How thoroughly researched and well designed the bot with the persona is, can be tested for how human the interaction with the cyber intelligence feels.
For instance, The Turing Test developed by Alan Turing in 1950 is a method to determine whether a machine is capable of thinking like a human being. In this test, a human evaluator converses with a human and a machine programmed to generate human-like responses. If the evaluator cannot reliably distinguish between the machine and the human, then the machine passes The Turing Test. In 1980, John Searle wrote a paper proposing “The Chinese Room” thought experiment. He argued that the Turing Test can be passed by simply manipulating symbols without having an actual understanding of those symbols. In the Chinese Room experiment, a human sits at a desk against a window with a tome placed on the desk. From time to time, a message in Chinese is dropped through the window onto the desk and the response to the message is suggested by the tome to the human. The human draws the exact character responses indicated by the tome and hands the response back through the window. The experiment outlined that an understanding of Chinese was not necessary in order to hand out correct responses as long as the tome could be consulted. He further argued that machines operate in this manner based on their programming, without actual understanding, and so they could not be described as “thinking beings” in the same sense as people can. Searle’s argument can be applied to tools such as Google Translate. Here, the translation is performed without understanding the language being translated and the output. The programmer programs the responses of the machine based on inputs. However, Google published an interesting research article last year on something called ‘Zero-Shot Translation’ which explains how it’s translation tool has learnt to translate into languages which hadn’t been programmed into the translation system’s original code. According to this research, the tool has invented a base language for translation which has enabled translation from a few languages to 103 languages in a matter of a decade.
On one hand, OpenAI is working on the artificial intelligence nonprofit lab founded by Elon Musk and Y Combinator president Sam Altman where they’re training AI bots to create their own language by trail and error. On another, Facebook AI Research Lab (FAIR) has been all over the news as Facebook researchers shut down it’s AI Systems because while the researchers were working on improving chat bots, the digital assistants had invented their own language to converse with each other and which wasn’t part of their initial programming. You can read the story here.
The advances in artificial intelligence and machine learning have shown that if systems are made with capabilities to understand and learn, then the systems can manipulate/change their initial variables of programming and can detect or forecast patterns not necessarily programmed into the system’s original design. This kind of artificial but true intelligence will not only guide actions of individuals but also take decisions and actions on behalf of those individuals realizing the dream of complete anticipatory design.
Tesla is building self-driving hardware into it’s cars. Tesla Autopilot can detect and avoid crashes through its forward collision warning system. This technology is not new, other cars such as ‘The Infiniti’ have intelligent breaking systems which when turned on would warn and slow down the car when required. The early forms of intelligent automobiles can be traced to a decade ago when cars had basis systems to control the switching on and off of lights, vipers and other isolated controls. How does this technology work? Autonomous cars use GPS, cameras, scanners, radars to detect obstacles and avoid collisions. A central system analyzes the data and accelerates or slows down the cars depending on this analysis. A system where cars can talk to each other through a central computer can tremendously improve on the current state of road traffic and safety. However, in a scenario where the central computer becomes intelligent enough to manipulate its initial programming or malfunctions leading to an accident on the road – who will be held accountable for the accident? Will it be the designer of the system, the programmer, the organization or the machine? How data analysis was performed to arrive at a decision or next course of action is still a ‘black box’ in such learning systems. Due to the current lack of transparency or clarity on the robustness of the technology, it will be difficult to trust AI unless it has been thoroughly tested and meanwhile constantly monitored.
Another industry that can be looked at when we talk about road safety is that of healthcare. One of the biggest user pain points in healthcare is when users have to fill lengthy forms online. In the healthcare domain, users are usually compelled to answer hundreds of questions to initiate a simple task such as consultation. AI, when allowed to penetrate these systems would possibly autofill or suggest answers thus making user’s time worthwhile. Through machine learning, our connected devices already talk to a bunch of apps, providing them realtime information about the users. This could also bring down the possibility of insurance fraud when intelligent systems already have data about users. Ofcourse, privacy has been an age old concern for users and it will play a major role in trusting intelligent devices that are collecting data and performing computations on that data.
The Banking & Financial Services Industry has been slow to adopt AI but the applications of this technology in the industry could be groundbreaking. The next level of recommendation engines would be engines that can take decisions on behalf of the users based on their past behavior patterns and preferences. For example, let’s say AI understands a user’s spending behavior and any anomaly in this behavior is monitored and controlled to reduce the impact of theft. One step further would be if intelligent banking assistants can accurately detect theft of cards and contact the authorities to take necessary actions. In such scenarios, the design of intelligence should be such that it can give the user some room to alter past behavior and preferences.
In summary, if the ability to think, understand and learn can be designed into machines – then we are talking about artificial intelligence which can make decisions and take actions on a user’s behalf, thereby alleviating decision fatigue.
One of the key factors in penetration of AI would be the level of trust the users place on upcoming scenarios. When mapping scenarios, the challenges of AI such as possible malfunction, privacy, spontaneity must be considered seriously and designed to accomodate.
While we continue to debate on the potential threat to employment due to AI, we cannot help but think how Princess Diana could have been alive and how we could have possibly avoided or mitigated the impact of 9/11attack if we had AI during those times. View Part 1