A variety of factors influence the operation of a well-designed AI (artificial intelligence) system, but these factors can sometimes carry biases that affect the results produced. To ensure the effects don’t pose a threat to the outcomes or performance of the system, it is important to arm ourselves with the capability to accurately identify them.
Source
Bhavini Kumari & Stuti Mazumdar - July 2023
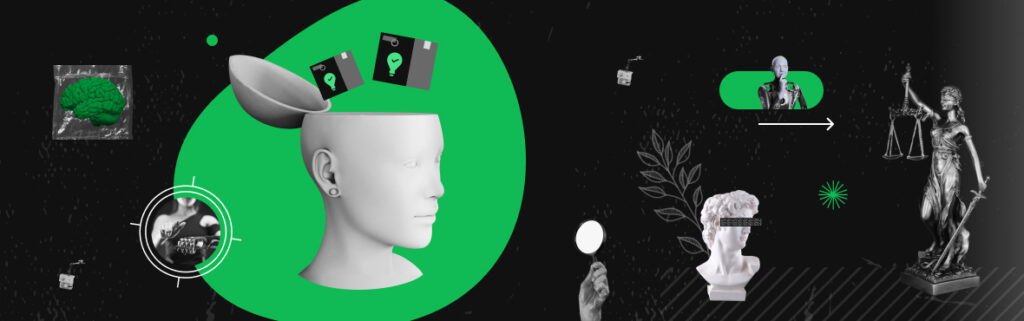
Our Responsibility Against AI Biases
Biases hurt and are unfair to those who are discriminated against, and it is the responsibility of all of us who run, build, and use AI systems to work against them. For example, Amazon stopped using a hiring algorithm that favored applicants based on specific words that appeared more frequently on men’s resumes. Amazon modified the programs to make them neutral toward these words.
Source
A lot can be done to eliminate biases in AI, such as staying current with AI research, establishing processes to mitigate biases, considering how humans and machines can collaborate to mitigate bias, and much more. Let us look at some methods for checking biases in AI systems below!
How to overcome AI biases
- Data should be preprocessed before being used to train AI systems to remove any bias. This allows the system to be fed only unbiased data, thus creating a better AI system.
- Another approach is to scan and process the AI system for biases. This means modifying some of the predictions made, to satisfy an arbitrary fairness constraint determined in advance.
- It is critical to predict domains that may be prone to unfair bias when implementing AI. AI developers must stay up to date to see how and where AI can improve justice.
- It demands the use of many tools and approaches capable of identifying potential bias sources and highlighting the data qualities that have the greatest influence on the results. Transparency regarding methods and measurements can assist onlookers in comprehending the actions taken to ensure fairness.
- Engaging in fact-based discussions about potential biases can help determine whether the proxies used previously are acceptable and how AI can help reveal long-standing prejudices that may have gone unreported.
- Explore how humans and machines can work best together in a variety of scenarios and application cases, and develop AI.
- Multidisciplinary participation in AI development processes, such as that of social scientists and ethicists, can aid in understanding the complexities of the system’s various applications and algorithms.
- Investing more in extensive research as well as allowing more data to be available for study is essential when considering potential risks and privacy concerns.
Witnessing The First Steps
Big players like Google, IBM, Microsoft, and OpenAI have all taken steps to address and mitigate AI biases. For example, Microsoft has created a toolkit called Fairlearn that supports developers in assessing and mitigating unfairness in machine learning models. Google has also released a set of guidelines and principles for ethical AI development and formed an external advisory council to provide diverse perspectives on AI-related challenges.
OpenAI acknowledged concerns about biases in their AI systems and intended to improve their performance. They built their models in two steps: pre-training and fine-tuning, but they recognize that they are still a work in progress.
IBM has emphasized the importance of fairness while being open about its efforts to reduce AI biases and increase transparency in AI systems.
“IBM is committed to advancing the fairness, transparency, and accountability of AI systems by developing novel technologies, tools, and best practices to mitigate bias and promote responsible AI deployment.” – Dario Gil, Director of IBM Research
Even if we take all steps to eliminate bias in AI, the system may harbor some prejudices. As a result, it is even more crucial to keep systems constantly updated and biases checked regularly.